New AI model helps detect and identify microplastics in wastewater
새로운 AI 모델은 폐수에서 미세 플라스틱을 감지하고 식별하는 데 도움
by Abhishyant Kidangoor on 16 January 2024
- A new model developed by researchers at the University of Waterloo in Canada uses advanced spectroscopy and artificial intelligence to identify the presence of microplastics in wastewater.
- 캐나다 워털루 대학교 연구진이 개발한 새로운 모델은 고급 분광학 및 인공 지능을 사용하여 폐수에서 미세플라스틱의 존재를 식별합니다.
- Researchers trained PlasticNet to detect microplastics based on how they absorb and transmit different wavelengths of light that they’re exposed to.
- 연구원들은 노출된 다양한 파장의 빛을 흡수하고 전달하는 방식을 기반으로 미세 플라스틱을 감지하도록 PlasticNet을 교육했습니다.
- The tool successfully classified 11 types of common plastics with an accuracy of more than 95%; it could potentially be used by wastewater treatment plants and food producers to identify microplastics.
- 이 도구는 95% 이상의 정확도로 11가지 유형의 일반 플라스틱을 성공적으로 분류했습니다. 이는 폐수 처리장과 식품 생산자가 미세 플라스틱을 식별하는 데 잠재적으로 사용될 수 있습니다.
- The team is currently working to make the model work faster and more efficiently, and to also streamline the process of gathering data.
- 팀은 현재 모델이 더 빠르고 효율적으로 작동하도록 만들고 데이터 수집 프로세스를 간소화하기 위해 노력하고 있습니다.
How do you identify microplastics in wastewater? When Wayne Parker started out researching the issue, the existing techniques were time-consuming and cumbersome. For a long time, optical or infrared microscopes were used to manually analyze and identify the tiny fragments of plastic waste in the water. However, differentiating microplastics from other substances took time and expertise.
“When we started looking at more advanced microscopy methods, we found that the analysis of the images that were being generated from those microscopes had some issues,” Parker, a professor of civil and environmental engineering at the University of Waterloo in Ontario, Canada, told Mongabay in a video interview. “We thought it could be improved upon by using more of a deep learning, AI-based approach.”
That led Parker to his colleague Alexander Wong, an expert in artificial intelligence and a professor in the systems design engineering department at the same university. Together, they worked with a PhD candidate to develop an image identification system that could potentially be used by wastewater treatment plants and food producers to identify the presence of microplastics and reduce the harm they pose to human health and the environment.
PlasticNet uses deep learning, a subset of artificial intelligence, to identify microplastics based on the signals they produce in response to light exposure. A study published by the team in the journal Environmental Pollution details how they trained the model to detect microplastics based on their interaction with different wavelengths of light. While the tool isn’t publicly available yet, it was shown to be able to identify microplastics accurately and rapidly. According to the study, “once trained with 8000+ spectra of virgin plastic, PlasticNet successfully classified 11 types of common plastic with accuracy higher than 95%.”
Early detection of microplastics — defined as pieces of plastic less than 5 millimeters (0.2 inches) in length — in wastewater is crucial because wastewater treatment plants are a significant source of microplastic pollution in marine environments. “The plastics themselves are a concern and there is concern about other kinds of pollutants that associate themselves with microplastics,” Parker said. “We wanted to look at the levels and quantities that would get discharged into rivers and lakes.”
폐수 속 미세플라스틱을 어떻게 식별하나요?
Wayne Parker가 이 문제를 연구하기 시작했을 때 기존 기술은 시간이 많이 걸리고 번거로웠습니다. 오랫동안 광학현미경이나 적외선 현미경을 사용하여 물 속의 작은 플라스틱 폐기물 조각을 수동으로 분석하고 식별했습니다. 그러나 미세플라스틱을 다른 물질과 구별하는 데에는 시간과 전문 지식이 필요했습니다.
캐나다 온타리오주 워털루대학교 토목환경공학과 교수인 파커(Parker)는 "보다 진보된 현미경 방법을 조사하기 시작했을 때 현미경에서 생성된 이미지를 분석하는 데 몇 가지 문제가 있다는 사실을 발견했습니다."라고 말했습니다. 비디오 인터뷰에서 Mongabay에게 말했습니다. "우리는 AI 기반 접근 방식인 딥 러닝을 더 많이 사용하면 개선될 수 있다고 생각했습니다."
그래서 Parker는 인공 지능 전문가이자 같은 대학의 시스템 설계 공학과 교수인 동료 Alexander Wong을 만나게 되었습니다. 그들은 함께 박사 과정 후보자와 협력하여 폐수 처리장 및 식품 생산업체에서 미세 플라스틱의 존재를 식별하고 인간 건강과 환경에 미치는 피해를 줄이기 위해 사용할 수 있는 이미지 식별 시스템을 개발했습니다.
PlasticNet은 인공 지능의 하위 집합인 딥 러닝을 사용하여 빛 노출에 반응하여 생성되는 신호를 기반으로 미세플라스틱을 식별합니다.
환경 오염(Environmental Pollution) 저널에 팀이 발표한 연구에서는 다양한 파장의 빛과의 상호 작용을 기반으로 미세플라스틱을 감지하도록 모델을 훈련한 방법을 자세히 설명합니다. 이 도구는 아직 공개되지 않았지만 미세플라스틱을 정확하고 빠르게 식별할 수 있는 것으로 나타났습니다.
연구에 따르면, "8000개 이상의 순수 플라스틱 스펙트럼으로 훈련을 받은 후 PlasticNet은 95%보다 높은 정확도로 11가지 유형의 일반 플라스틱을 성공적으로 분류했습니다."
폐수 처리장은 해양 환경에서 미세플라스틱 오염의 중요한 원인이기 때문에 폐수에서 길이가 5밀리미터(0.2인치) 미만의 플라스틱 조각으로 정의되는 미세플라스틱을 조기에 검출하는 것이 중요합니다.
Parker는 “플라스틱 자체가 우려 사항이며 미세 플라스틱과 관련된 다른 종류의 오염 물질에 대한 우려가 있습니다.”라고 말했습니다. "우리는 강과 호수로 배출되는 수준과 양을 살펴보고 싶었습니다."
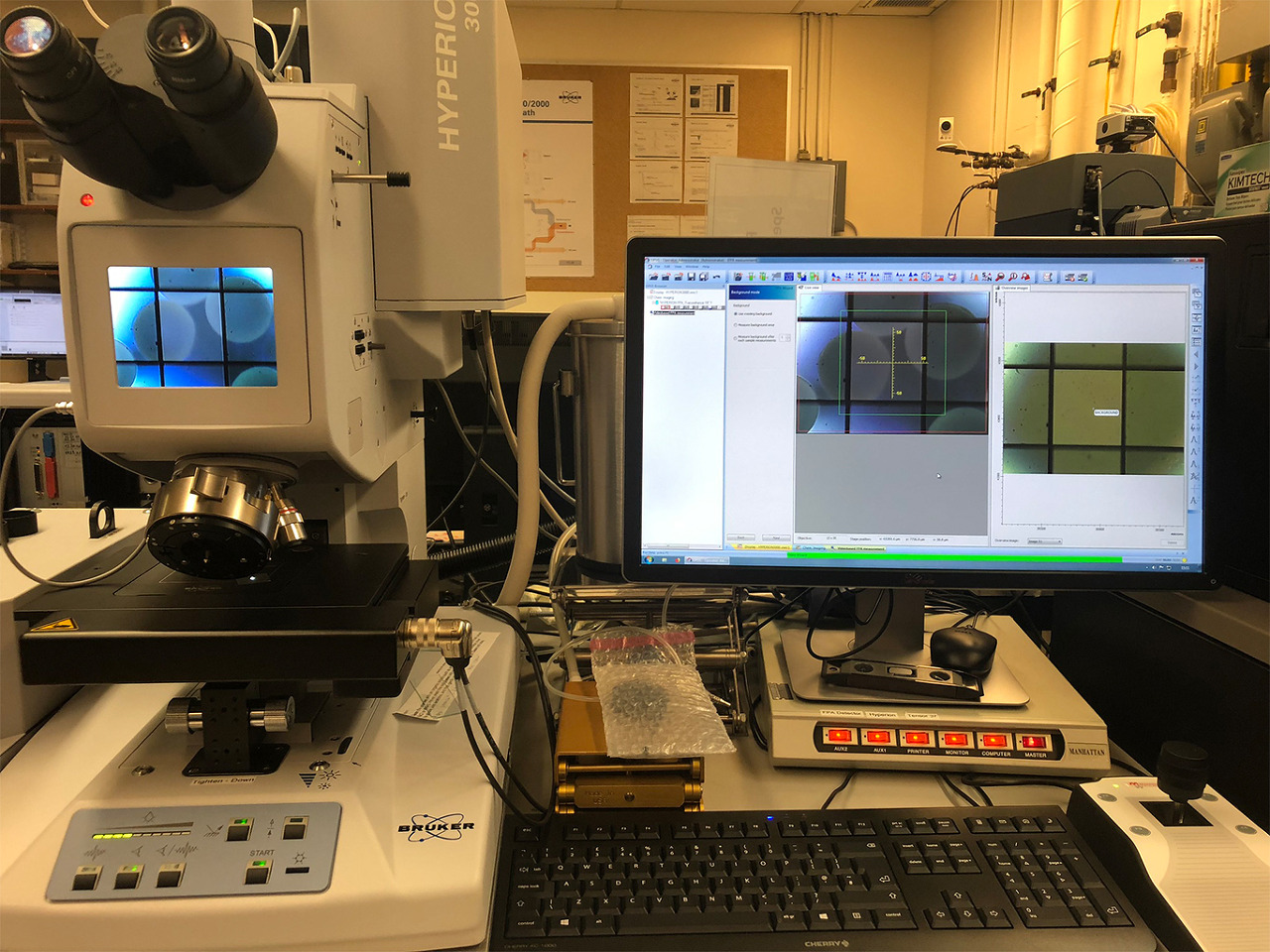
PlasticNet uses advanced spectroscopy and AI to detect microplastics in wastewater. Image courtesy of the University of Waterloo. PlasticNet은 고급 분광학 및 AI를 사용하여 폐수에서 미세플라스틱을 감지합니다. 이미지 제공: 워털루 대학교
To begin with, the team used an advanced spectroscopy technique in which they shone a light on the water. Different kinds of plastics absorb and transmit light in different wavelengths. “You look at how the light is transmitted through the plastic and then do a scan of wavelengths and look at what the corresponding signature is,” Parker said. “That signature can give you an insight into the type of plastic that you’re working with.”
The model was then trained in small, progressive steps. It was first trained with commonly used plastics. As the training progressed, Parker and his team introduced more complex plastics to account for the fact that most commercial plastics aren’t just pure polymers, but also contain additives, like dyes that give them color or other chemicals that enhance properties such as ductility and heat resistance. “Those, of course, impact the transmission of light,” he said. “We wanted to evaluate whether our method could still identify the base polymer that was making up the plastic even when these other kinds of additives were there and modifying the signature that was generated from the microscope.”
As increasingly complex plastics of different shapes, sizes and thickness were introduced into the training package, the team observed the model’s accuracy and efficiency improve. When they compared their approach to the traditional methods of identifying microplastics, Parker said, “it was about 50% faster and more accurate.”
Parker, however, said the model can still be improved and that it requires further training with more complex plastics over a period of months. He said he also wants to look into optimizing the image-gathering process, which tends to be slow and often comprises highly dense information.
“We should certainly look at whether we could be more efficient in terms of the quantity of data that we need to try and get,” Parker said. “Just the amount of data that we need to do the analysis and not more than is necessary, so that we could expedite some of the steps along the way to make the overall analysis more efficient.”
우선 연구팀은 물에 빛을 비추는 첨단 #분광학 기술을 사용했다. 다양한 종류의 플라스틱은 다양한 파장의 빛을 흡수하고 전달합니다. Parker는 “빛이 플라스틱을 통해 어떻게 투과되는지 살펴본 다음 파장을 스캔하여 해당 신호가 무엇인지 확인합니다.”라고 말했습니다. "이 서명을 통해 작업 중인 플라스틱 유형에 대한 통찰력을 얻을 수 있습니다."
그런 다음 모델은 작고 점진적인 단계로 훈련되었습니다. 처음에는 일반적으로 사용되는 플라스틱을 사용하여 훈련되었습니다. 훈련이 진행됨에 따라 Parker와 그의 팀은 대부분의 상업용 플라스틱이 단순한 폴리머가 아니라 색상을 부여하는 염료나 연성 및 내구성과 같은 특성을 향상시키는 기타 화학 물질과 같은 첨가제도 포함하고 있다는 사실을 설명하기 위해 더 복잡한 플라스틱을 도입했습니다. 내열성. “물론 이는 빛의 전달에 영향을 미칩니다.”라고 그는 말했습니다. "우리는 다른 종류의 첨가제가 존재하고 현미경에서 생성된 특성을 수정하는 경우에도 우리의 방법으로 플라스틱을 구성하는 기본 폴리머를 식별할 수 있는지 평가하고 싶었습니다."
다양한 모양, 크기 및 두께의 점점 더 복잡한 플라스틱이 교육 패키지에 도입됨에 따라 팀은 모델의 정확성과 효율성이 향상되는 것을 관찰했습니다. Parker는 그들의 접근 방식을 미세플라스틱을 식별하는 전통적인 방법과 비교했을 때 "약 50% 더 빠르고 정확했습니다"라고 말했습니다.
그러나 Parker는 모델이 여전히 개선될 수 있으며 몇 달에 걸쳐 더 복잡한 플라스틱에 대한 추가 교육이 필요하다고 말했습니다. 그는 또한 속도가 느리고 종종 고밀도 정보로 구성되는 이미지 수집 프로세스를 최적화하는 방법도 조사하고 싶다고 말했습니다.
Parker는 “우리가 시도하고 확보해야 하는 데이터의 양 측면에서 더 효율적일 수 있는지 확실히 살펴봐야 합니다.”라고 말했습니다. "분석을 수행하는 데 필요한 데이터의 양은 필요한 것보다 많지 않으므로 전체 분석을 보다 효율적으로 만드는 과정에서 일부 단계를 가속화할 수 있습니다."
Banner image: Plastic waste in water. Image by 7inchs via Pexels (Public domain).
Abhishyant Kidangoor is a staff writer at Mongabay. Find him on 𝕏 @AbhishyantPK.
Citation:
Zhu, Z., Parker, W., & Wong, A. (2023). Leveraging deep learning for automatic recognition of microplastics (MPs) via focal plane array (FPA) micro-FT-IR imaging. Environmental Pollution, 337, 122548. doi:10.1016/j.envpol.2023.122548
New AI model helps detect and identify microplastics in wastewater
How do you identify microplastics in wastewater? When Wayne Parker started out researching the issue, the existing techniques were time-consuming and cumbersome. For a long time, optical or infrared microscopes were used to manually analyze and identify th
news.mongabay.com
https://www.sciencedirect.com/science/article/abs/pii/S0269749123015506?via%3Dihub
Leveraging deep learning for automatic recognition of microplastics (MPs) via focal plane array (FPA) micro-FT-IR imaging
The fast and accurate identification of MPs in environmental samples is essential for the understanding of the fate and transport of MPs in ecosystems…
www.sciencedirect.com